Terracotta is an experiment-builder (currently in development) integrated with a learning management system (LMS), allowing a teacher or researcher to randomly assign different versions of online learning activities to students, collect informed consent, and export deidentified study data. Terracotta is a portmanteau of Tool for Educational Research with RAndomized COnTrolled TriAls, and is designed to lower the technical and methodological barriers to conducting more rigorous and responsible education research.
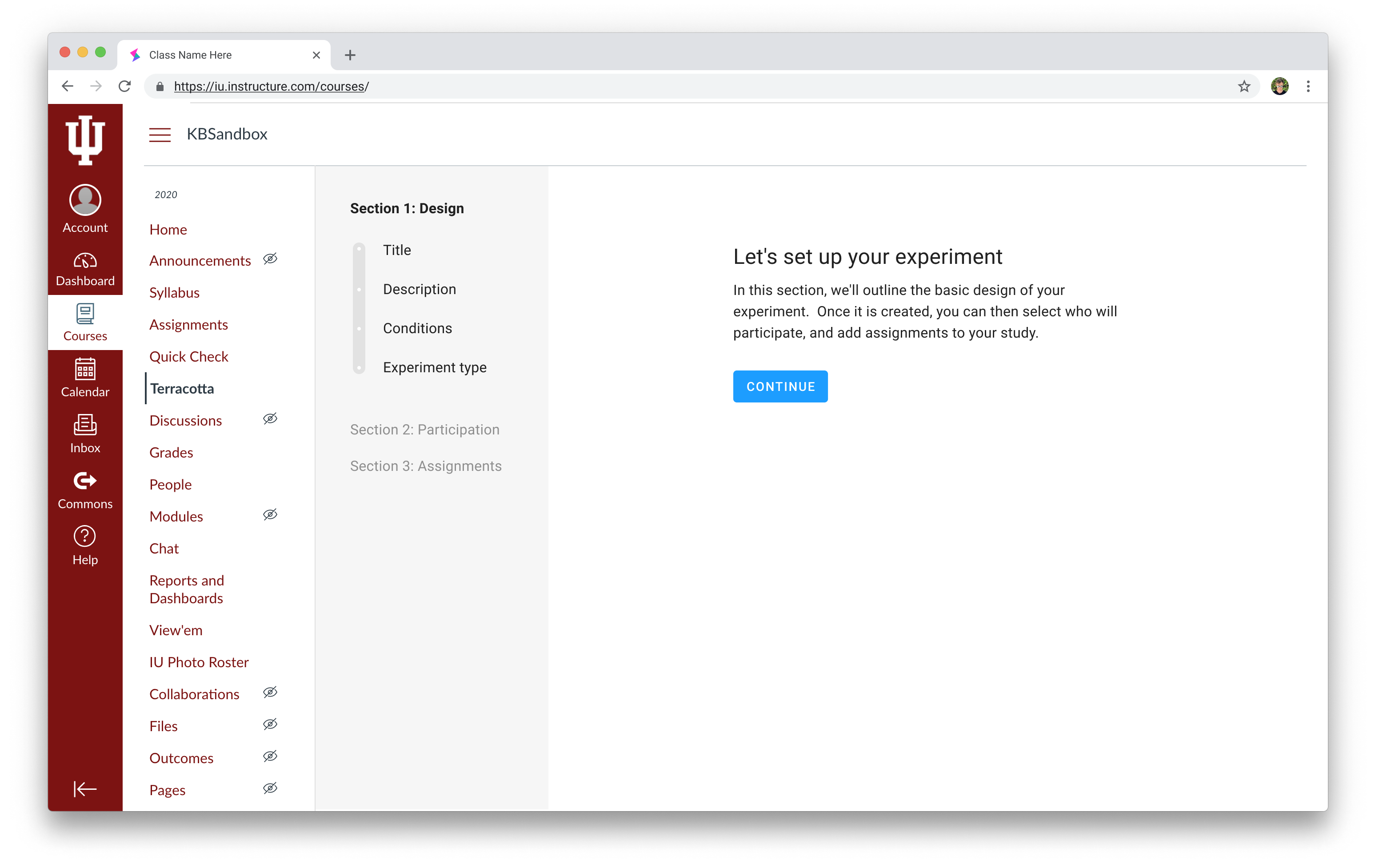
Terracotta is currently under development at Indiana University, with support from Schmidt Futures, a philanthropic initiative co-founded by Eric and Wendy Schmidt, and in collaboration with the Learning Agency Lab.
Why do we need an experiment builder?
To reform and improve education in earnest, we need research that conclusively and precisely identifies the instructional strategies that are most effective at improving outcomes for different learners and in different settings — what works for whom in what contexts. To test whether an instructional practice exerts a causal influence on an outcome measure, the most straightforward and compelling research method is to conduct an experiment. But despite their benefits, experiments are also exceptionally difficult and expensive to carry out in education settings (Levin, 2005; Slavin, 2002; Sullivan, 2011).
Perhaps due to this difficulty, rigor in educational research has been trending downward. Roughly 79% of published STEM education interventions fail to provide strong evidence in support of their claims (Henderson et al., 2011), and the number of experimental educational research studies has been in steady decline, from 47% of published education studies in 1983, to 40% in 1994, to 26% in 2004, even while the prevalence of causal claims is increasing (Hsieh et al., 2005; Robinson et al., 2007). When education research studies do succeed in making strong causal claims, the replicability of these findings are disappointingly low (Makel & Plucker, 2014; Schneider, 2018).
To reverse these trends, we need to eliminate the barriers that prevent teachers and researchers from conducting experimental research on instructional practices.
Can an experiment-builder improve the ethics of classroom research?
Every teacher, in every classroom, is actively experimenting on their students. Every time a teacher develops a new lesson, makes a planning decision, or tries a new way to motivate a disengaged student, the teacher is, in a sense, carrying out an experiment. These “experiments” are viewed as positive features of a teacher’s professional development, and we generally assume that these new experimental “treatments” represent innovations over default instruction. What’s obviously missing in these examples is random assignment of students to treatment conditions, and therefore these are also missing a compelling way of knowing whether the new strategy worked.
By our view, it is even more ethical to conduct experiments when these experiments are done carefully and rigorously, using random assignment to different treatment conditions (Motz et al., 2018). This is because randomized experiments enable a teacher or researcher to infer whether the new strategy causes improvements without bias. In the absence of random assignment to treatment conditions, a teacher is stuck making comparisons based on subjective reflection, or based on confounded comparisons with other cohorts; this can cause ineffective strategies to appear to have worked, or effective strategies to be missed.
Even so, experiments conducted recklessly can clearly be unethical, so one of Terracotta’s central goals is to streamline and normalize the features of responsible, ethical experimentation. For example, students should be assigned to experimental variants of assignments (rather than deprived no-treatment controls), whenever possible research should favor within-subject crossover designs where all students receive all treatments (eliminating any bias due to condition assignment), students should be empowered to provide informed consent, and student data should be deidentified prior to export and analysis. Each of these standards is easily achievable using standard web technology, but no tool currently exists that meets these standards. That’s where Terracotta comes in.
What will Terracotta do?
Based on years of experience working through the challenges of implementing ethical and rigorous experiments in authentic online learning environments, Terracotta automates the methodological features that are fundamental to responsible experimental research (but that are particularly difficult to implement without dedicated software), seamlessly integrating experimental treatments within the existing ecosystem of online learning activities. For example:
- Within-Subject Research Designs. A particularly effective way to ensure balanced, equitable access to experimental treatments is to have all students receive all treatment conditions, except different groups receive these treatments in different orders. This kind of within-subject crossover design will be implementable with a single click.
- Random Assignment that Automatically Tracks Enrollment Changes. If students add the class late, new enrollments will be repeatedly monitored and manually assigned to a group to achieve balance and ensure access to experimental manipulations.
- Informed Consent that Conceals Responses from the Instructor. Informed consent is a cornerstone in the ethical conduct of research. This choice should be protected from the teacher until grades are finalized, because many review boards are sensitive that a teacher is in a position to coerce students to participate. Terracotta will collect consent responses in a way that is temporarily hidden from the instructor.
- Mapping Outcomes to Assignments. An experiment examines how different treatments affect relevant outcomes, such as learning outcomes (e.g., exam scores) or behavioral outcomes (e.g., attendance). Terracotta will include a feature where instructors can import, identify in the gradebook, or manually enter outcome data into Terracotta following each treatment event (for within-subject crossover designs) or at the end of an experiment (for between-subject designs).
- Export of De-identified Data from Consenting Participants. At the end of the term, Terracotta will allow an export of all study data, with student identifiers replaced with random codes, and with non-consenting participants removed. This export set will include: (1) condition assignments; (2) scores on manipulated learning activities; (2) granular clickstream data for interactions with Terracotta assignments; and (3) outcomes data as entered by the instructor. By joining these data, de-identifying it, and scrubbing non-consenting participants, Terracotta can prepare a data export that is shareable with research collaborators (includes no personally identifiable information) and that meets ethical requirements (only includes data from consenting students).
What’s the overarching goal of Terracotta?
The goal is to enable more rigorous, more responsible experimental education research. But we don’t simply need more experiments — if this were the case, we could conveniently conduct behind-the-scenes experiments within existing learning technologies. Instead, we need more experiments that are carried out in classes where it might have otherwise been inconvenient to conduct an experiment using existing technologies, to better-understand the context-dependency of learning theory (Motz & Carvalho, 2019). We need grand-scale experiments that are systematically distributed across scores of classrooms to test how different experimental implementations moderate the effects of instructional practices (Fyfe et al., 2019). We need teachers and researchers to test the effects of recommended instructional practices with replication studies, so that we can improve the credibility of education research and identify the boundary conditions of current theory (Makel et al., 2019). We need teachers to be empowered to test their latent knowledge of what works in their classrooms, through experimental research that is more inclusive of practitioners (Schneider & Garg, 2020). To achieve these ends, we don’t simply need to make existing educational tools compatible with experimental research, we need to make an experimental research tool that is compatible with education settings.
References
Fyfe, E., de Leeuw, J., Carvalho, P., Goldstone, R., Sherman, J., and Motz, B. (2019). ManyClasses 1: Assessing the generalizable effect of immediate versus delayed feedback across many college classes. PsyArXiv, https://psyarxiv.com/4mvyh/
Henderson, C., Beach, A., Finkelstein, N. (2011). Facilitating change in undergraduate STEM instructional practices: An analytic review of the literature. Journal of Research in Science Teaching, 48, 952–984. doi:10.1002/tea.20439
Hsieh, P., Acee, T., Chung, W.-H., Hsieh, Y.-P., Kim, H., Thomas, G.D., You, J., Levin, J.R., and Robinson, D.H. (2005). Is educational intervention research on the decline? Journal of Educational Psychology, 97, 523-529. doi:10.1037/0022-0663.97.4.523
Levin, J.R. (2005). Randomized classroom trials on trial. In G.D. Phye, D.H. Robinson, and J.R. Levin (Eds.), Empirical Methods for Evaluating Educational Interventions, pp. 3–27. Burlington: Academic Press. doi:10.1016/B978-012554257-9/50002-4
Makel, M.C., and Plucker, J.A. (2014). Facts are more important than novelty: Replication in the education sciences. Educational Researcher, 43(6), 304-316. doi:10.3102/0013189X14545513
Makel, M.C., Smith, K.N., McBee, M.T., Peters, S.J., & Miller, E.M. (2019). A path to greater credibility: Large-scale collaborative education research. AERA Open, 5(4), doi:10.1177/2332858419891963
Motz, B.A., Carvalho, P.F., de Leeuw, J.R., & Goldstone, R.L. (2018). Embedding experiments: Staking causal inference in authentic educational contexts. Journal of Learning Analytics, 5(2), 47-59. doi:10.18608/jla.2018.52.4
Motz, B.A. and Carvalho, P.F. (2019). Not whether, but where: Scaling-up how we think about effects and relationships in natural educational contexts. In Companion Proceedings of the 9th International Conference on Learning Analytics & Knowledge (LAK’19). doi:10.13140/RG.2.2.30825.34407
Robinson, D.H., Levin, J.R., Thomas, G.D., Pituch, K.A., and Vaughn, S. (2007) The incidence of “causal” statements in teaching-and-learning research journals. Am Educ Res J. 2007;44: 400–413. doi:10.3102/0002831207302174
Schneider, M. (2018). A more systematic approach to replicating research. Message from the Director, Institute for Education Sciences (IES), December 17, 2018. https://ies.ed.gov/director/remarks/12-17-2018.asp
Schneider, M. & Garg, K. (2020). Medical researchers find cures by conducting many studies and failing fast. We need to do the same for education. The 74 Million. https://www.the74million.org/article/schneider-garg-medical-researchers-find-cures-by-conducting-many-studies-and-failing-fast-we-need-to-do-the-same-for-education/
Slavin, R.E. (2002). Evidence-based education policies: Transforming educational practice and research. Education Researcher, 31, 15–21. doi:10.3102/0013189X031007015Sullivan G.M. (2011). Getting off the “gold standard”: randomized controlled trials and education research. Journal of Graduate Medical Education, 3, 285-289. doi:10.4300/JGME-D-11-00147.1